TAILOR - Foundations of Trustworthy AI - Integrating Reasoning, Learning and Optimization
Short Title: TAILOR
Project Areas: Data, Content and Media
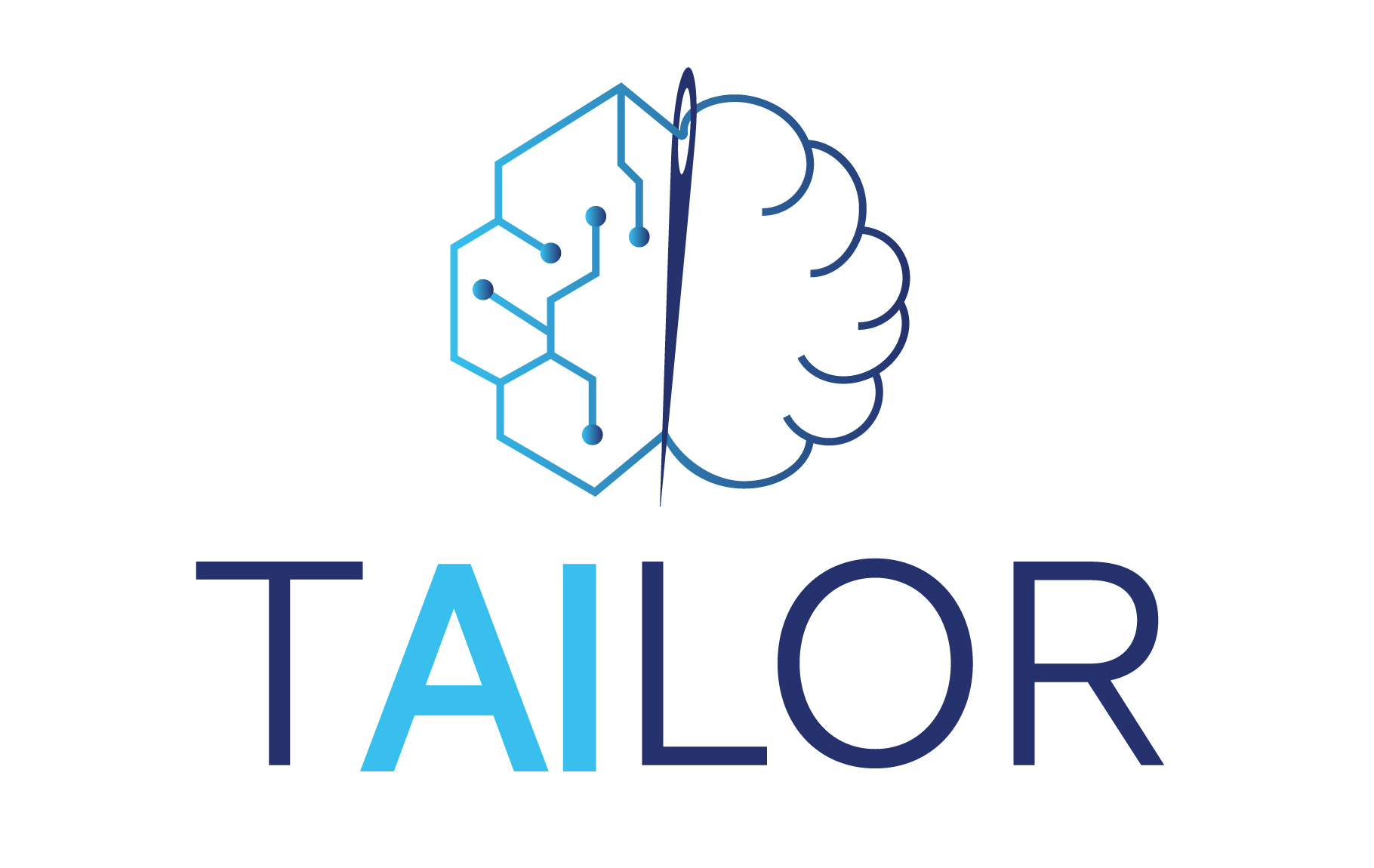
Staff
Riccardo Albertoni, Silvia Biasotti, Ettore Lanzarone, Michela Spagnuolo, Silvia Zuffi
Year of start/end
2020 / 2024
PDGP CODE
DIT.AD004.115.006
Tag
metadata management | Metadata standard | optimization | reproducibility | Uncertainty | W3C
Tailor web siteActivity
The research activity will study the continuous interdisciplinary dialogue for investigating the methods and methodologies to design, develop, assess, and enhance systems that fully implement Trustworthy AI, aiming to create AI systems that incorporate trustworthiness by design. Different dimensions pertain to Trustworthy AI: explainability, safety and robustness, fairness, accountability, privacy, and sustainability. The overall mission for Trustworthy AI is to combine the various dimensions in the TAILOR research and innovation roadmap.
IMATI participate in the project as part of a larger CNR partnership that involves different institutes. In the project context, IMATI will investigate existing metadata models created to support the documentation of AI Systems. It aims to enhance the interoperability and reusability of AI components and fill the identified gaps in accountability and reproducibility by leveraging some of the contributed W3C metadata vocabularies. Among the W3C metadata vocabularies, DCAT and DQV will be considered as they offer a starting point to standardize and make interoperable the representations and results of the Trustworthy-AI metrics identified by TAILOR.
Moreover, the research activity will investigate promising integrations between AI and optimization approaches in order to improve the description of the uncertain parameters in the real-life application of optimization techniques. With respect to classical approaches where the uncertainty is first modelled (e.g., with AI approaches) and the resulting stochastic coefficients are inserted in the optimization method, our approach will aim at fully integrating them in order to describe the uncertainty in the light of the final optimization goal.
HighLight
TAILOR - Foundations of Trustworthy AI - Integrating Reasoning, Learning and Optimization
Linked Projects
Linked Products
- 2023, Journal article
Quality of word and concept embeddings in targetted biomedical domains
Salvatore Giancani, Riccardo Albertoni, Chiara Eva Catalano