STATISTICAL MACHINE LEARNING FOR EXPOSURE DEVELOPMENT
Short Title: SMILE
Principal Investigator: Elisa Varini
Project Areas: Matematica Applicata
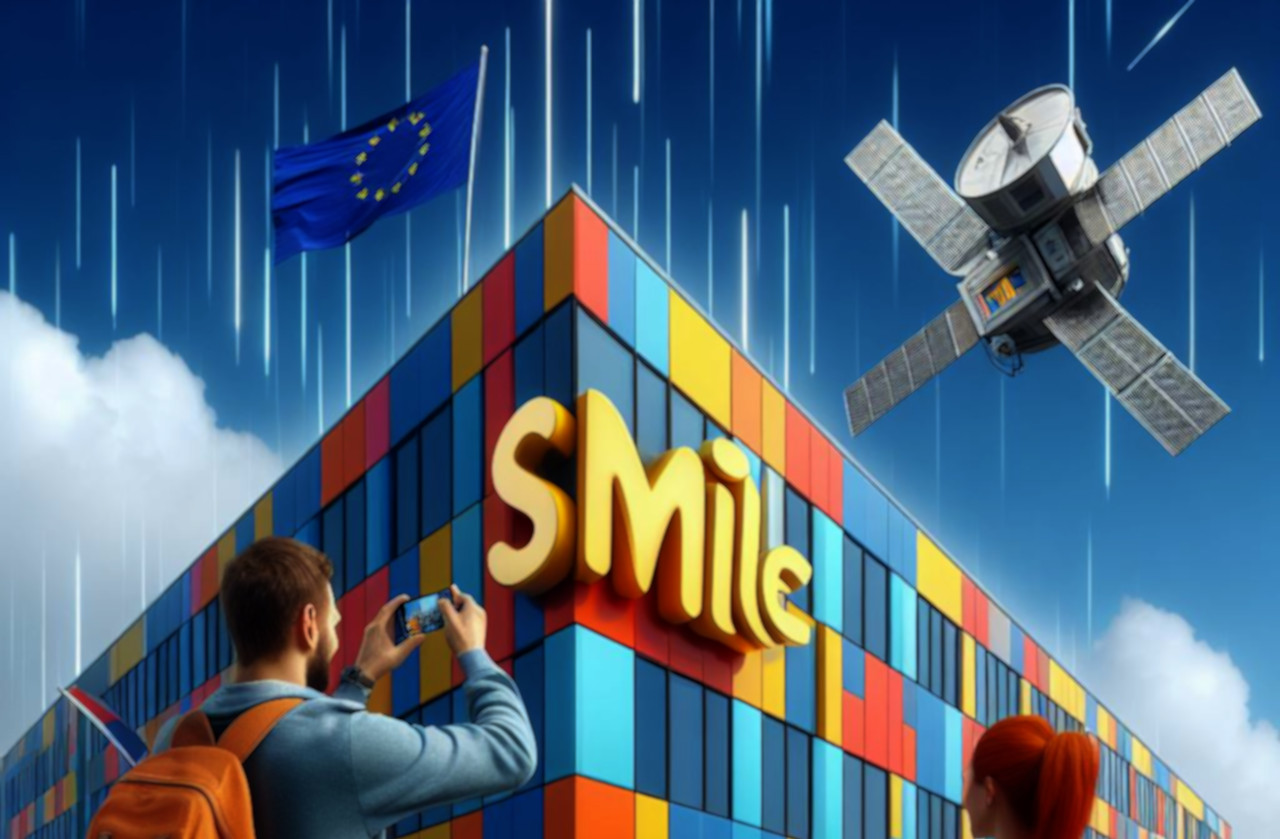
Coordinator
Chiara Scaini, OGS
Staff
Maria Teresa Artese, Isabella Gagliardi
Funding Body
Progetto PRIN 2022 PNRR promosso dal MUR e finanziato dall'Unione Europea - NextGenerationEU
Year of start/end
2023 / 2025
PDGP CODE
PRR.PN007.001
Project Web SiteActivity
SMILE is a Project of Significant National Interest (Progetto di Rilevante Interesse Nazionale - PRIN) funded by the National Recovery and Resilience Plan (Recovery Plan or NRRP) from the European Union - Next Generation EU and coordinated by the National Institute of Oceanography and Experimental Geophysics - OGS, with the participation of the University of Florence (UNIFI), the University of Milan-Bicocca (UNIMIB), and the Institute of Applied Mathematics and Information Technology of the National Research Council (CNR-IMATI)
SMILE (Statistical Machine Learning for Exposure development) aims at developing new methods for exposure assessment combining multiple data sources. In particular, it will leverage crowdsourced data collected by citizens using the CEDAS (Buildings CEnsus for seismic DAmage ASsessment) approach, developed by OGS in recent years. CEDAS will be complemented considering exposure to multiple hazards (e.g. landslides, tsunamis). Also, other data types will be used, including remote sensing images of different types and resolutions. Machine learning tools will be tested and their potential will be demonstrated in order to automatically classify buildings in selected urbanized areas, based on different sets of inputs.
HighLight
The SMILE project aims at exploiting and further developing the most advanced ML technologies and visualization techniques to contribute to addressing current challenges in Disaster Risk Reduction (DRR). In particular, it will demonstrate that exposure development can rely on crowdsourced data from citizens and on remote sensing products, and how these inputs can be combined with existing information. The procedure will be automated thanks to machine learning techniques that will be tested and implemented.